News
Members
Publications
Software / Data
Job offers
Images / Videos
Collaborations
Conferences
Lab meetings: "Les partages de midi"
Practical information
Members Area
Next conferences we are in …

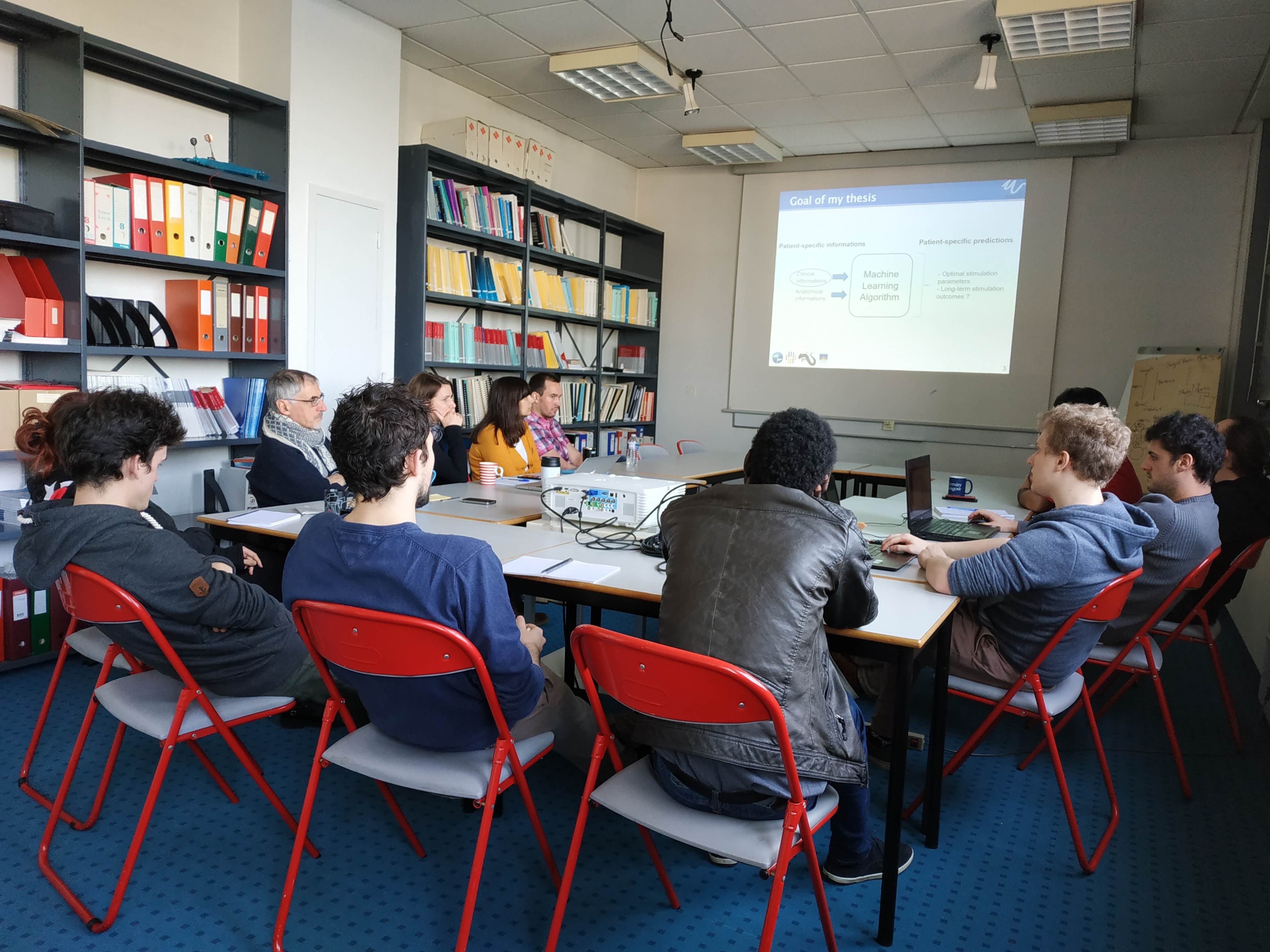
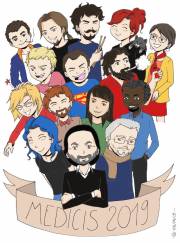
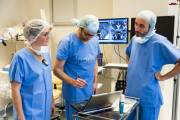
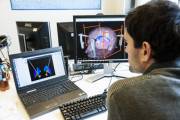
This is an old revision of the document!
Pierre Jannin, Xavier Morandi, Laurent Riffaud, Claire Haegelen, David Bouget, Frederic Monge, Tiziano D'albis, Gregory Laheurte
Data fusion in medicine is the process of extracting and integrating relevant information from different sources of data and modalities to help the decision-making process. In this aim, innovative data fusion approaches for building surgically relevant specific and generic models will be studied.
These last 20 years, a lot of research teams addressed computer assisted surgical support as the display of patient images during planning and surgery using navigation systems. They (we also) focused on the image registration aspects in order to build pre operative patient-specific models from the patient’s own multimodal medical images, available for planning and displayed during surgery. Additionally, approaches have been studied for updating the patient specific models according to intraoperative anatomical deformations due to surgery, based on intraoperative imaging such as stereoscopic reconstructions of surface of the operative field, ultrasound (US) imaging, or intraoperative MR. Despite this important amount of work, image guided surgery (IGS) and more generally computer assisted surgical (CAS) systems have not demonstrated their full clinical added value. One reason is that surgical support was rarely considered as a full surgical decision support system relying on relevant data, information, and knowledge about the patient and the surgical process, in numeric and symbolic form, extracted from patient data and large multisite-data repositories. Consequently, IGS and CAS systems are sub optimally used by the surgeon when taking his surgical decision.
The concept of surgical process models (SPM), we introduced about 10 years ago, is now emerging as a key element of the operating room of the future, assuming that an optimal surgical support system needs to have an explicit understanding of the surgical process. Surgical process models are descriptions of the surgical or interventional procedure formalized and represented into structured forms. Different granularity levels of the procedure may be studied from a high-level objective-based list of main surgical or interventional phases, to a list of the physical actions performed by the operators and recorded during surgery, to the spatial description of each physical action and tool. For the two firsts, a classical representation of surgical process consists in a sequential list of n-uplets describing the surgical action by a verb, the operator performing the action, the surgical tool, the targeted anatomical, pathological and/or functional structure(s), the body part concerned by the performance of this action, the starting and ending times of the action, the needed information for performing this action, and some additional attributes characterizing the action. We developed initial tools and methods for acquiring, displaying, storing, and analyzing such surgical process models. We used such approach for studying surgical or interventional related differences between different populations of patients and surgeons. This initial work has several limitations that need to be addressed in order to fully benefit from these models. First, there is a need for developing methods allowing to compare and register different surgical process models in order to build models characterizing similar surgical cases. These methods should rely on the sequential dimension on the models. Second, observer-based acquisition of surgical process models restricts the added value of this approach. With automatic acquisition approaches, SPMs will be used on-line in the OR for optimal surgical assistance.
In this axis, we want to overcome main limitations outlined above by studying data fusion methods for building and analyzing specific and generic models. We will address the specificities of data fusion for two main aspects of patient data: data representing the patient itself (such as multimodal images and clinical scores) and data representing the surgical and interventional procedure planned or performed for this specific patient. Specific models will be built from registration of different views of the same phenomenon (i.e. of the same patient), whereas generic models will be built from registration of data and information from different patients coming from a homogeneous population. In a long term, we aim at developing methods for generating predictive models of the outcome and of the surgical and interventional procedure from learning from patient-specific models. For instance, predicting the motor improvement and the clinical side effects after deep brain stimulation for Parkinson disease or predicting the sequence of surgical steps and options in case of cervical spinal surgery, such as Anterior Cervical Discectomy and Fusion.