News
Members
Publications
Software / Data
Job offers
Images / Videos
Collaborations
Conferences
Lab meetings: "Les partages de midi"
Practical information
Members Area
Next conferences we are in …

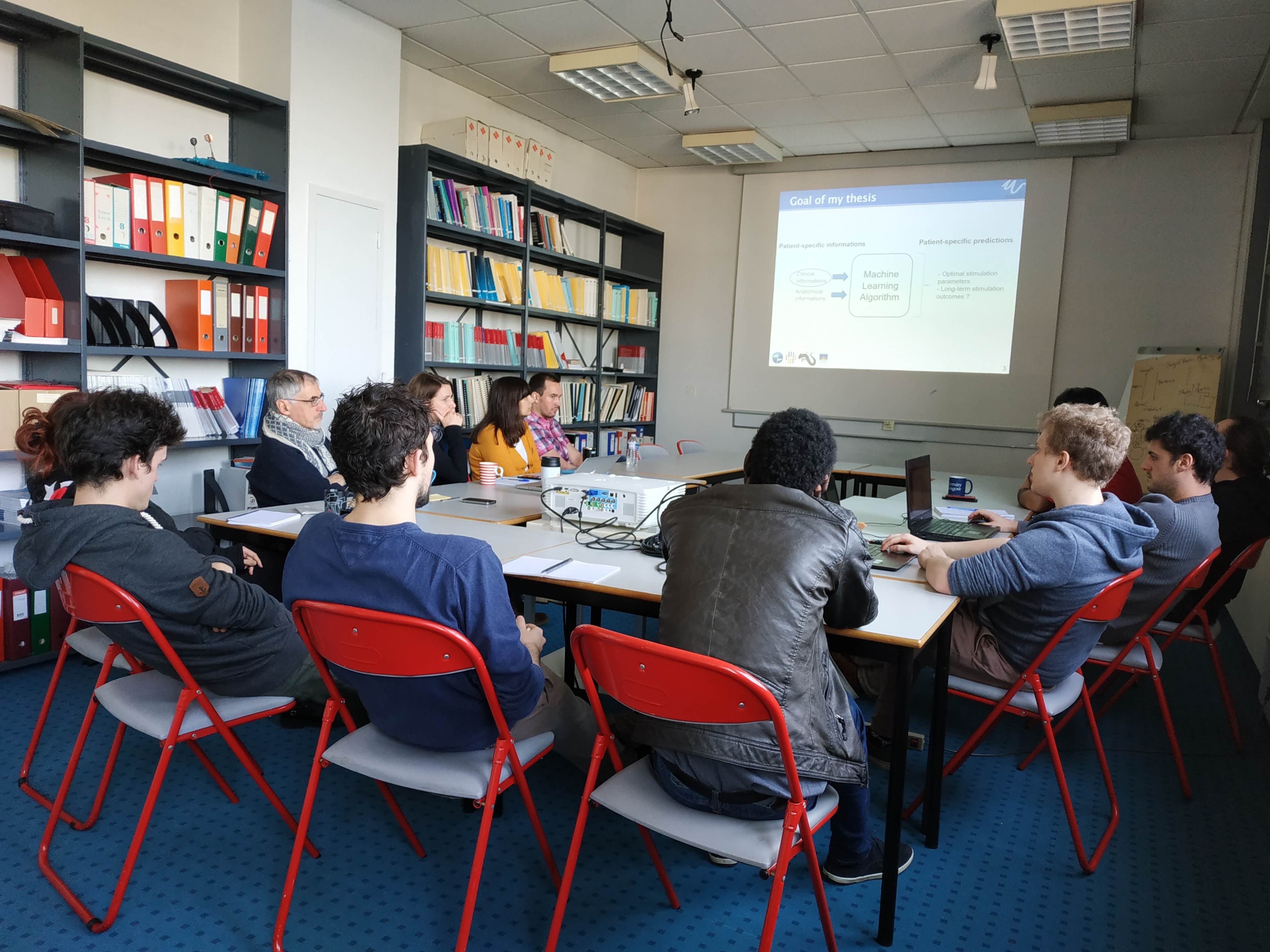
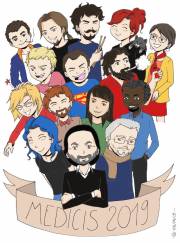
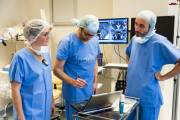
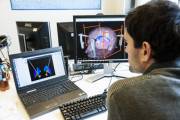
This shows you the differences between two versions of the page.
Both sides previous revision Previous revision | Last revision Both sides next revision | ||
news:ipcai2016award [2016/06/30 15:09] odergach |
news:ipcai2016award [2016/06/30 15:10] odergach |
||
---|---|---|---|
Line 8: | Line 8: | ||
**Automatic data-driven real-time segmentation and recognition of surgical workflow** \\ //O. Dergachyova, D. Bouget, A. Huaulmé, X. Morandi, P. Jannin// | **Automatic data-driven real-time segmentation and recognition of surgical workflow** \\ //O. Dergachyova, D. Bouget, A. Huaulmé, X. Morandi, P. Jannin// | ||
- | **ABSTRACT** | + | **ABSTRACT** \\ |
Purpose - With the intention of extending the perception and action of surgical staff inside the operating room, the medical community has expressed a growing interest towards context-aware systems. Requiring an accurate identification of the surgical workflow, such systems make use of data from a diverse set of available sensors. In this paper, we propose a fully data-driven and real-time method for segmentation and recognition of surgical phases using a combination of video data and instrument usage signals, exploiting no prior knowledge. We also introduce new validation metrics for assessment of workflow detection. | Purpose - With the intention of extending the perception and action of surgical staff inside the operating room, the medical community has expressed a growing interest towards context-aware systems. Requiring an accurate identification of the surgical workflow, such systems make use of data from a diverse set of available sensors. In this paper, we propose a fully data-driven and real-time method for segmentation and recognition of surgical phases using a combination of video data and instrument usage signals, exploiting no prior knowledge. We also introduce new validation metrics for assessment of workflow detection. | ||
Methods - The segmentation and recognition are based on a four-stage process. Firstly, during the learning time, a Surgical Process Model is automatically constructed from data annotations to guide the following process. Secondly, data samples are described using a combination of low-level visual cues and instrument information. Then, in the third stage, these descriptions are employed to train a set of AdaBoost classifiers capable of distinguishing one surgical phase from others. Finally, AdaBoost responses are used as input to a Hidden semi-Markov Model in order to obtain a final decision. | Methods - The segmentation and recognition are based on a four-stage process. Firstly, during the learning time, a Surgical Process Model is automatically constructed from data annotations to guide the following process. Secondly, data samples are described using a combination of low-level visual cues and instrument information. Then, in the third stage, these descriptions are employed to train a set of AdaBoost classifiers capable of distinguishing one surgical phase from others. Finally, AdaBoost responses are used as input to a Hidden semi-Markov Model in order to obtain a final decision. |