News
Members
Publications
Software / Data
Job offers
Images / Videos
Collaborations
Conferences
Lab meetings: "Les partages de midi"
Practical information
Members Area
Next conferences we are in …

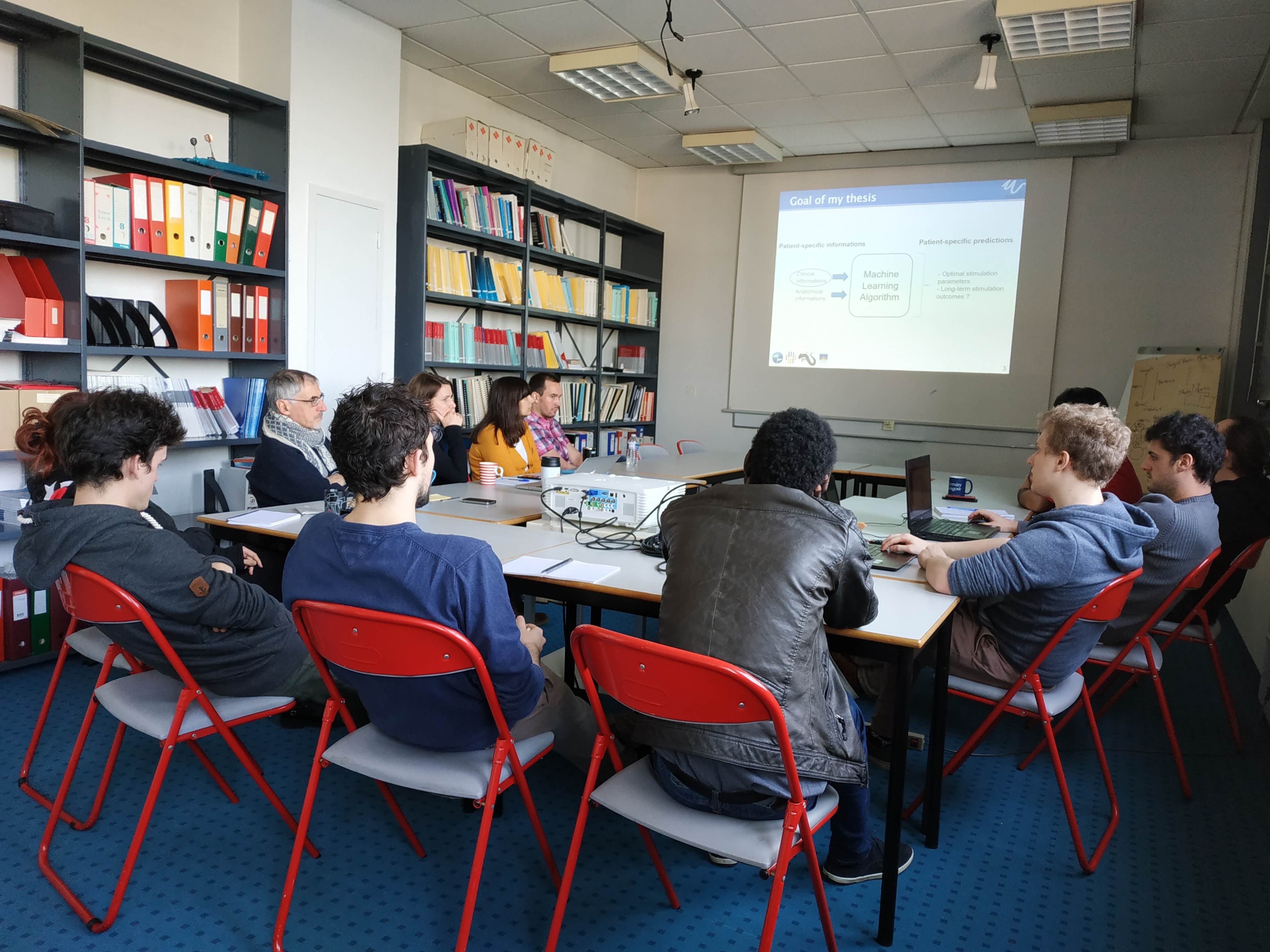
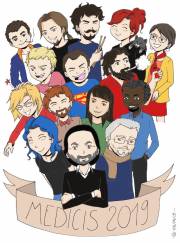
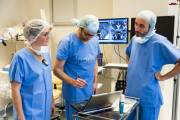
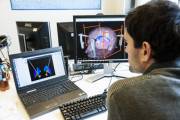
—-
Sir Michael Brady FRS FREng FMedsci
Emeritus Professor of Oncological Imaging, University of Oxford
Executive Chairman: National Consortium on Intelligent Medical Imaging
Founder Chairman: Perspectum Diagnostics, ScreenPoint Medical
Founder Director: Volpara Health Technologies, Mirada Medical
Chairman: Optellum
(Membre Étranger de l’Académie des Sciences)
Title:
Quantitative and Intelligent Imaging for Clinical Decision Support
Abstract:
We discuss a number of important developments in CARS primarily by reference to innovations in some of the medical image analysis companies of which I am a Founder. First, image analysis can be quantitative, each pixel measuring a physical quantity. We first illustrate this by quantitative MRI of the liver, measuring proton density fat fraction; iron content; and fibroinflammation (units of time). This is applied to (non-alcoholic) fatty liver disease, steatohepatitis (NASH), and therapeutic interventions, both measuring the effect of anti-NASH drugs and supporting liver surgery. Then, we show how breast density may be measured and applied to estimate x-ray dose in mammography. Second, image analysis can be intelligent based on methods developed in AI and Machine Learning (a branch of AI). We illustrate this both in MRI analysis of the liver and in a decision support system for mammography. This offers opportunities in work flow and we show how the combination of all radiologists working with Transpara decision support software can out-perform either working individually. Finally, we discuss some of the strengths and limitations of machine learning applied to medical imaging.
Chief Medical Officer
American College of Radiology Data Science Institute
Diagnostic Radiology
Grandview Medical Center
Birmingham, Alabama USA
Title:
Fostering A Strong Ecosystem For Artificial Intelligence In Medical Imaging
Abstract:
Fueled by the ever-increasing amount of data generated by the healthcare system applications for artificial intelligence in healthcare, especially within diagnostic imaging, are rapidly proliferating. Currently, no well-defined framework exists for determining how great ideas for AI algorithms in healthcare will advance from development to integrated clinical practice. Healthcare stakeholders including physicians, patients, medical societies, hospital systems, software developers, the health information technology industry and governmental regulatory agencies all comprise a community that will need to function as an ecosystem system in order for AI algorithms to be deployed, monitored, and improved in widespread clinical practice. Radiologists can play an important role in promoting this AI ecosystem by delineating structured AI use cases for diagnostic imaging and standardizing data elements and workflow integration interfaces. By developing structured AI use cases based on the needs of the physician community, radiologists and radiology specialty societies can assist developers in creating the tools that will advance the practice of medicine. If these use cases specify how datasets for algorithm training, testing and validation can be developed as well as specifying parameters for clinical integration and pathways for assessing algorithm performance in clinical practice, the likelihood of bringing safe and effective algorithms to clinical practice will increase dramatically. The development of an active AI ecosystem will facilitate the development and deployment of AI tools for healthcare that will help physicians solve medicine’s important problems.