News
Members
Publications
Software / Data
Job offers
Images / Videos
Collaborations
Conferences
Lab meetings: "Les partages de midi"
Practical information
Members Area
Next conferences we are in …

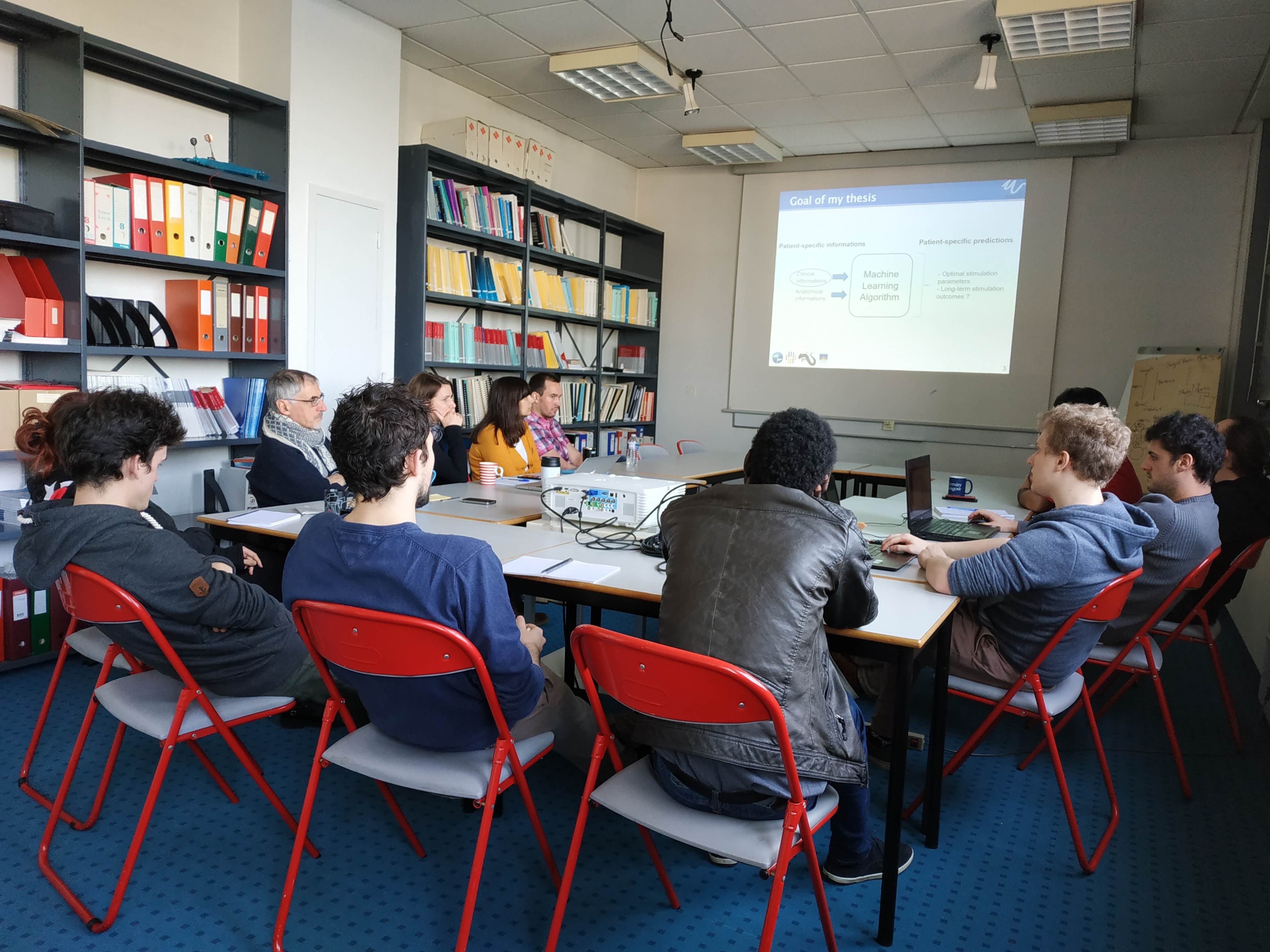
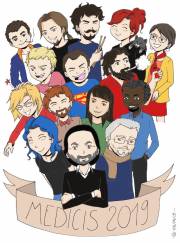
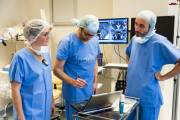
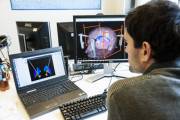
Nous avons le plaisir de vous annoncer que deux papiers ont été acceptés à la conférence SPIE Medical Imaging, qui aura lieu à San Diego (Etats-Unis) du 27 Février au 3 Mars 2016. Ils seront présentés par Frédéric Monge (poster) et Clément Baumgarten (oral).
Détail des papiers présentés ci-dessous :
Partition Based Acquisition Model for Speed-Up Navigated Beta-probe Surface Imaging
Frederic Monge, Dzoshkun I. Shakir, Nassir Navab and Pierre Jannin,
ABSTRACT: Although gross total resection in low-grade glioma surgery leads to a better patient outcome, the in-vivo control of resection borders remains challenging. For this purpose, navigated beta-probe systems combined with FDG radiotracer, relying on activity distribution surface estimation, have been proposed to generate reconstructed images. The clinical relevance has been outlined by early studies where intraoperative functional information are leveraged although inducing low spatial resolution in reconstruction. To improve reconstruction quality, acquisition models have been suggested. They involve the definition of attenuation matrix for designing radiation detection physics. Yet, they require high computational power for an efficient intraoperative usage. To address the problem, we propose a new acquisition model called Partition Model (PM) considering an existing model where coefficients of the matrix are taken from a look-up table (LUT). Our model is based upon the division of the LUT into particular homogeneous values for assigning attenuation coefficients. To validate our model, we ran multiple experiments using in- vitro data sets. Three spatial configurations of grown cancer cells using Petri dish as phantom, simulating tumors and peri-tumoral tissues, have been acquired. We compared our acquisition model with the off-the-shelf LUT and the raw method. Acquisition models outperformed the raw method in term of tumor contrast (7.97:1 mean T:B) but with an impossibility of real time usage. Both acquisition models reached the same detection performances with references (0.8 mean AUC and 0.77 mean NCC). Yet PM slightly improves the mean tumor contrast up to 10.1:1 vs 9.9:1 with the LUT model and more importantly reduces the mean computation time by 7.5%.
Image-guided preoperative prediction of pyramidal tract side effect in deep brain stimulation
C. Baumgartena, Y. Zhaoa, C. Malrainc, P. Sauleauc, P. Jannina,b, C. Haegelena,
ABSTRACT Deep brain stimulation of the medial globus pallidus is a surgical procedure for treating patients suffering from Parkinson’s disease. Its therapeutic effect may be limited by the presence of pyramidal tract side effect (PTSE). PTSE is a contraction time-locked to the stimulation when the current spreading reaches the motor fibers of the pyramidal tract within the internal capsule. The lack of side-effect predictive model leads the neurologist to secure an optimal electrode placement by iterating clinical testing on an awake patient during the surgical procedure. The objective of the study was to propose a preoperative predictive model of PTSE. A machine learning based method called PyMAN (for Pyramidal tract side effect Model based on Artificial Neural network) that accounted for the current of the stimulation, the 3D electrode coordinates and the angle of the trajectory, was designed to predict the occurence of PTSE. Ten patients implanted in the medial globus pallidus have been tested by a clinician to create a labeled dataset of the stimulation parameters that trigger PTSE. The kappa index value between the data predicted by PyMAN and the labeled data was .78. Further evaluation studies are desirable to confirm whether PyMAN could be a reliable tool for assisting the surgeon to prevent PTSE during the preoperative planning.